Artificial intelligence pioneer says humans must teach machines
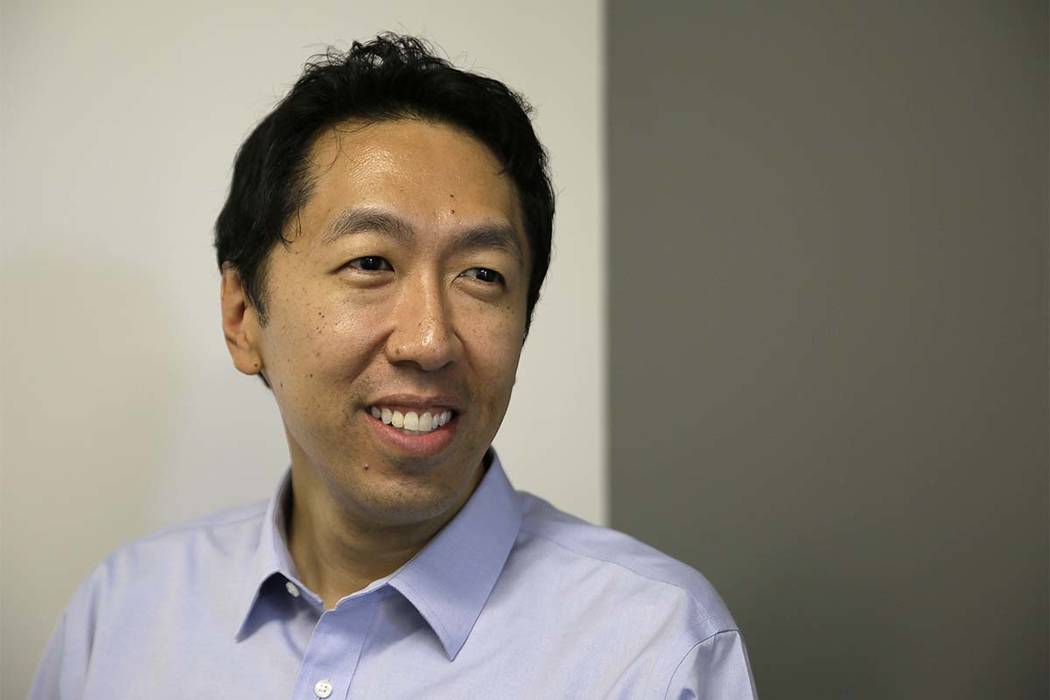
PALO ALTO, Calif. — Andrew Ng has led teams at Google and Baidu that have gone on to create self-learning computer programs used by hundreds of millions of people, including email spam filters and touch-screen keyboards that make typing easier by predicting what you might want to say next.
As a way to get machines to learn without supervision, he has trained them to recognize cats in YouTube videos without being told what cats were. And he revolutionized this field, known as artificial intelligence, by adopting graphics chips meant for video games.
To push the boundaries of artificial intelligence further, one of the world’s most renowned researchers in the field says many more humans need to get involved. So his focus now is on teaching the next generation of AI specialists to teach the machines.
Nearly 2 million people around the globe have taken Ng’s online course on machine learning. In his videos, the lanky, 6-foot-1 Briton of Hong Kong and Singaporean upbringing speaks with a difficult-to-place accent. He often tries to get students comfortable with mind-boggling concepts by acknowledging up front, in essence, that “hey, this stuff is tough.”
Ng sees AI as a way to “free humanity from repetitive mental drudgery.” He has said he sees AI changing virtually every industry, and any task that takes less than a second of thought will eventually be done by machines. He once said famously that the only job that might not be changed is his hairdresser’s — to which a friend of his responded that in fact, she could get a robot to do his hair.
At the end of a 90-minute interview in his sparse office in Palo Alto, California, he reveals what’s partially behind his ambition.
“Life is shockingly short,” the 41-year-old computer scientist says, swiveling his laptop into view. He’s calculated in a Chrome browser window how many days we have from birth to death: a little more than 27,000. “I don’t want to waste that many days.”
Building brains as a teen
An upstart programmer by age 6, Ng learned coding early from his father, a medical doctor who tried to program a computer to diagnose patients using data. “At his urging,” Ng says, he fiddled with these concepts on his home computer. At age 16, he wrote a program to calculate trigonometric functions like sine and cosine using a “neural network” — the core computing engine of artificial intelligence modeled on the human brain.
“It seemed really amazing that you could write a few lines of code and have it learn to do interesting things,” he said.
After graduating high school from Singapore’s Raffles Institution, Ng made the rounds of Carnegie Mellon, MIT and Berkeley before taking up residence as a professor at Stanford University.
There, he taught robotic helicopters to do aerial acrobatics after being trained by an expert pilot. The work was “inspiring and exciting,” recalls Pieter Abbeel, then one of Ng’s doctoral students and now a computer scientist at Berkeley.
Abbeel says he once crashed a $10,000 helicopter drone, but Ng brushed it off. “Andrew was always like, ‘If these things are too simple, everybody else could do them.’”
The mark of Ng
Ng’s standout AI work involved finding a new way to supercharge neural networks using chips most often found in video-game machines.
Until then, computer scientists had mostly relied on general-purpose processors — like the Intel chips that still run many PCs. Such chips can handle only a few computing tasks simultaneously, but make up for it with blazing speed. Neural networks, however, work much better if they can run thousands of calculations simultaneously. That turned out to be a task eminently suited for a different class of chips called graphics processing units, or GPUs.
So when graphics chip maker Nvidia opened up its GPUs for general purposes beyond video games in 2007, Ng jumped on the technology. His Stanford team began publishing papers on the technique a year later, speeding up machine learning by as much as 70 times.
Geoffrey Hinton, whose University of Toronto team wowed peers by using a neural network to win the prestigious ImageNet competition in 2012, credits Ng with persuading him to use the technique. That win spawned a flurry of copycats, giving birth to the rise of modern AI.
“Several different people suggested using GPUs,” Hinton says by email. But the work by Ng’s team, he says, “was what convinced me.”
Teaching how to teach computers
Ng’s fascination with AI was paralleled by a desire to share his knowledge with students. As online education took off earlier this decade, Ng discovered a natural outlet.
His “Machine Learning” course, which kicked off Stanford’s online learning program alongside two other courses in 2011, immediately signed up 100,000 people without any marketing effort.
A year later, he co-founded the online-learning startup Coursera. More recently, he left his high-profile job at Baidu to launch deeplearning.ai , a startup that produces AI-training courses.
Every time he’s started something big, whether it’s Coursera, the Google Brain deep learning unit, or Baidu’s AI lab, he has left once he felt the teams he has built can carry on without him.
“Then you go, ‘Great. It’s thriving with or without me,’” says Ng, who continues to teach at Stanford while working in private industry.
For Ng, one of his next challenges might include having a child with his roboticist wife, Carol Reiley. “I wish we knew how children (or even a pet dog) learns,” Ng says in an email follow-up. “None of us today know how to get computers to learn with the speed and flexibility of a child.”